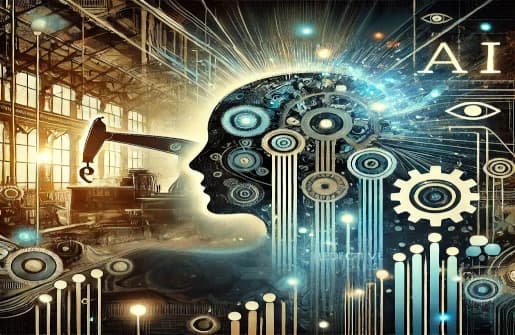
How Large Language Models can Impact Predictive Maintenance in Manufacturing Industries
Dr. Subalalitha CN
Apr 30, 2024
In the realm of manufacturing, where every minute of downtime can equate to significant losses, the need for predictive maintenance has never been more critical. Imagine a manufacturing facility specializing in precision engineering components, relying heavily on Computer Numerical Control (CNC) machining centers for production. These CNC machines are critical assets, responsible for shaping raw materials into intricate parts with high accuracy and efficiency. Any unplanned downtime or malfunctions in these machines can lead to costly production delays and quality issues, impacting the company’s bottom line and customer satisfaction.
The traditional maintenance approach employed by the manufacturing facility involves periodic inspections and scheduled maintenance tasks based on predefined intervals or equipment runtime. However, this reactive strategy is susceptible to unexpected failures, as it fails to account for variations in machine usage, environmental factors, and wear-and-tear patterns. The challenge is to transition from reactive maintenance to a predictive maintenance paradigm, leveraging data-driven insights to anticipate and prevent CNC machine failures proactively. However, with the advent of large language models (LLMs) powered by artificial intelligence, a transformative shift is occurring in how manufacturing industries approach maintenance.
Predictive maintenance, facilitated by LLMs, is a proactive approach that leverages data analytics and machine learning algorithms to predict equipment failures before they occur. By analyzing vast amounts of data collected from sensors, equipment logs, and historical maintenance records, LLMs can forecast potential issues, allowing manufacturers to take preemptive action, minimize downtime, and optimize maintenance schedules. Let’s delve deeper into how LLMs are revolutionizing predictive maintenance in manufacturing industries.
1. Data Integration and Analysis
One of the primary strengths of LLMs in predictive maintenance lies in their ability to process and interpret diverse datasets. Manufacturing environments generate a plethora of data from various sources, including IoT sensors, equipment telemetry, maintenance logs, and production records. LLMs excel in integrating these disparate data streams, identifying patterns, and extracting meaningful insights. Through natural language processing (NLP) capabilities, LLMs can comprehend unstructured data such as maintenance reports, equipment manuals, and technician notes. This enables them to discern subtle indicators of potential failures, even in complex machinery where traditional rule-based systems may fall short. By continuously learning from new data inputs, LLMs improve their predictive accuracy over time, enhancing the reliability of maintenance forecasts.
2. Condition Monitoring and Anomaly Detection
Predictive maintenance relies heavily on real-time condition monitoring and early anomaly detection. LLMs play a crucial role in this aspect by continuously monitoring equipment performance metrics and identifying deviations from normal operating conditions. By establishing baseline parameters for equipment health, LLMs can flag anomalies that may indicate impending failures or degradation.
Furthermore, LLMs can correlate multiple factors contributing to equipment health, including environmental conditions, production variables, and maintenance history. This holistic approach enables more nuanced predictions, where the interplay of various factors is taken into account. By distinguishing between benign fluctuations and critical abnormalities, LLMs empower manufacturers to prioritize maintenance efforts effectively and allocate resources efficiently.
3. Predictive Modeling and Decision Support
At the core of predictive maintenance is the development of robust predictive models that forecast equipment failures with high accuracy. LLMs leverage advanced machine learning techniques, including deep learning algorithms, recurrent neural networks, and transformer architectures, to build predictive models tailored to specific manufacturing processes and equipment types.
These models not only predict the likelihood of failures but also provide valuable insights into the root causes and potential consequences of malfunctions. By simulating different maintenance scenarios and estimating their impact on production schedules and resource utilization, LLMs enable informed decision-making by maintenance planners and operations managers. This proactive approach minimizes unplanned downtime, reduces maintenance costs, and enhances overall operational efficiency.
4. Continuous Improvement and Adaptation
The dynamic nature of manufacturing environments necessitates adaptive maintenance strategies that can evolve in response to changing conditions. LLMs excel in this regard by facilitating continuous improvement through iterative learning cycles. By analyzing feedback from maintenance actions and performance outcomes, LLMs refine their predictive models, incorporating new insights and refining their understanding of equipment behavior.
Moreover, LLMs can adapt to variations in operating conditions, equipment configurations, and production demands, ensuring that predictive maintenance remains effective across diverse scenarios. This adaptability is particularly valuable in industries with complex supply chains, fluctuating demand patterns, or seasonal production cycles, where traditional maintenance approaches may struggle to cope with variability.
Large language models are ushering in a new era of predictive maintenance in manufacturing industries, empowering organizations to proactively manage their assets, optimize productivity, and mitigate risks. By harnessing the power of artificial intelligence, data analytics, and machine learning, LLMs enable manufacturers to transition from reactive, schedule-based maintenance to proactive, data-driven strategies.
As LLM technology continues to advance, driven by ongoing research and innovation, its potential to revolutionize predictive maintenance will only grow. By embracing these transformative capabilities, manufacturing industries can stay ahead of the curve, ensuring operational resilience, competitiveness, and sustainability in an increasingly dynamic global marketplace.
Please write to teamfl@firstlanguage.in if you would like to empower your organization with LLM solutions. For details, visit FirstLanguage Technologies